File:DeepInsight method to transform non-image data to 2D image for convolutional neural network architecture.pdf
From Wikimedia Commons, the free media repository
Jump to navigation
Jump to search
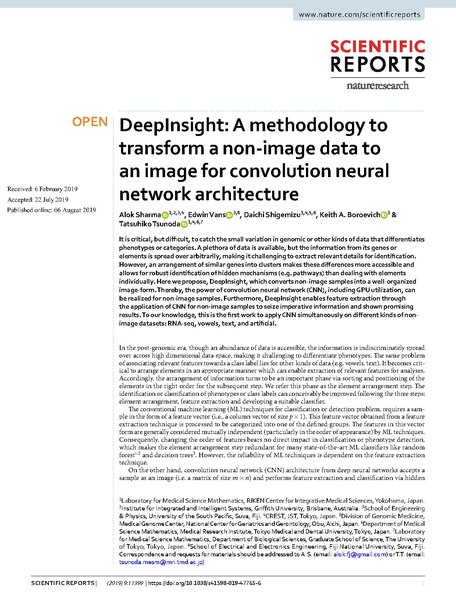
Size of this JPG preview of this PDF file: 456 × 600 pixels. Other resolutions: 182 × 240 pixels | 365 × 480 pixels | 584 × 768 pixels | 1,239 × 1,629 pixels.
Original file (1,239 × 1,629 pixels, file size: 1.78 MB, MIME type: application/pdf, 7 pages)
File information
Structured data
Captions
Captions
This paper, DeepInsight, describes a unique method of transforming a tabular or non-image data to an image form for CNN architecture. This method has been applied in various fields of research and promising results are obtained.
Summary[edit]
DescriptionDeepInsight method to transform non-image data to 2D image for convolutional neural network architecture.pdf |
English: It is critical, but difficult, to catch the small variation in genomic or other kinds of data that differentiates phenotypes or categories. A plethora of data is available, but the information from its genes or elements is spread over arbitrarily, making it challenging to extract relevant details for identification. However, an arrangement of similar genes into clusters makes these differences more accessible and allows for robust identification of hidden mechanisms (e.g. pathways) than dealing with elements individually. Here we propose, DeepInsight, which converts non-image samples into a well-organized image-form. Thereby, the power of convolution neural network (CNN), including GPU utilization, can be realized for non-image samples. Furthermore, DeepInsight enables feature extraction through the application of CNN for non-image samples to seize imperative information and shown promising results. To our knowledge, this is the first work to apply CNN simultaneously on different kinds of nonimage
datasets: RNA-seq, vowels, text, and artificial.
日本語: ゲノム解析で使われるような、表現型や種別における小さな変動を検出することは重要であり難しいタスクです。大量のデータが利用可能な状態であっても、その遺伝子または要素からの情報はランダムに広がっているため、識別のために関連する特徴を抽出することは困難です。 このような場合、類似の要素をクラスターに配置すると、これらの特徴抽出がしやすくなり要素を個別に処理するよりも隠れたメカニズム(経路など)を確実に識別できます。 本講演では、非画像サンプルをよく整理された画像形式に変換するDeepInsight法を提案します。 これにより、GPUの利用を含む畳み込みニューラルネットワーク(CNN)を非画像サンプルにも適用できるようになり、必須の情報と有望な結果を示すための特徴抽出を可能にします。 私たちの知る限り、これは、CNNをさまざまな種類の非画像データセット(RNAシーケンス、母音、テキスト、および人工)に同時に適用した、初めての研究です。 |
Date | |
Source | Own work |
Author | Alok Sharma |
Licensing[edit]
I, the copyright holder of this work, hereby publish it under the following license:



This file is licensed under the Creative Commons Attribution-Share Alike 4.0 International license.
- You are free:
- to share – to copy, distribute and transmit the work
- to remix – to adapt the work
- Under the following conditions:
- attribution – You must give appropriate credit, provide a link to the license, and indicate if changes were made. You may do so in any reasonable manner, but not in any way that suggests the licensor endorses you or your use.
- share alike – If you remix, transform, or build upon the material, you must distribute your contributions under the same or compatible license as the original.
File history
Click on a date/time to view the file as it appeared at that time.
Date/Time | Thumbnail | Dimensions | User | Comment | |
---|---|---|---|---|---|
current | 02:19, 1 July 2021 | ![]() | 1,239 × 1,629, 7 pages (1.78 MB) | Alok.fiji (talk | contribs) | Uploaded own work with UploadWizard |
You cannot overwrite this file.
File usage on Commons
The following page uses this file:
Metadata
This file contains additional information such as Exif metadata which may have been added by the digital camera, scanner, or software program used to create or digitize it. If the file has been modified from its original state, some details such as the timestamp may not fully reflect those of the original file. The timestamp is only as accurate as the clock in the camera, and it may be completely wrong.
Publisher | Springer US |
---|---|
Image title |
|
Short title |
|
Software used | Springer |
File change date and time | 14:51, 31 July 2019 |
Date and time of digitizing | 04:26, 31 July 2019 |
Date metadata was last modified | 14:51, 31 July 2019 |
Copyright status | Copyrighted |
Identifier | doi:10.1038/s41598-019-47765-6 |
Conversion program | iText® 5.3.5 ©2000-2012 1T3XT BVBA (AGPL-version) |
Encrypted | no |
Page size | 595.276 x 782.362 pts |
Version of PDF format | 1.4 |